During the summer of the COVID-19 pandemic, I was lucky enough to do research with Ohio State University on modeling and analyzing epidemics. The full paper has been published in SIAM Undergraduate Research Online Journal. Below is the abstract for the paper.
COVID-19 epidemics in many parts of the United States and the world have shown unexpected shifts from exponential to linear growth in the number of daily new cases. Epidemics on configuration model networks typically produce exponential growth, while epidemics on lattices produce linear growth. We explore a network-based epidemic model that interpolates between lattice-like and configuration model networks while keeping the degree distribution and basic reproduction number ($R_0$) constant. This model starts with nodes assigned random locations in a unit square and connected to their nearest neighbors. A proportion $p$ of the edges are disconnected and reconnected in a configuration model subnetwork. As $p$ increases, we observe a shift from linear to exponential growth. Realistic human contact networks involve many local interactions and fewer long-distance interactions, so social distancing affects both the effective reproduction number $R_t$ and the proportion of long-distance connections in the network. While the impact of changes in $R_t$ is well-understood, far less is understood about the effect of more subtle changes in network structure. Our analysis indicates that the threshold between linear and exponential growth may occur even with a small percentage of reconfigured edges. Additionally, the number of total infected individuals in an epidemic substantially increases around this threshold even when $R_0$ remains constant. This study reveals that implementing and relaxing social distancing restrictions can have more complex and dramatic effects on epidemic dynamics than previously thought.
To read more, go to https://doi.org/10.1137/20s1368227
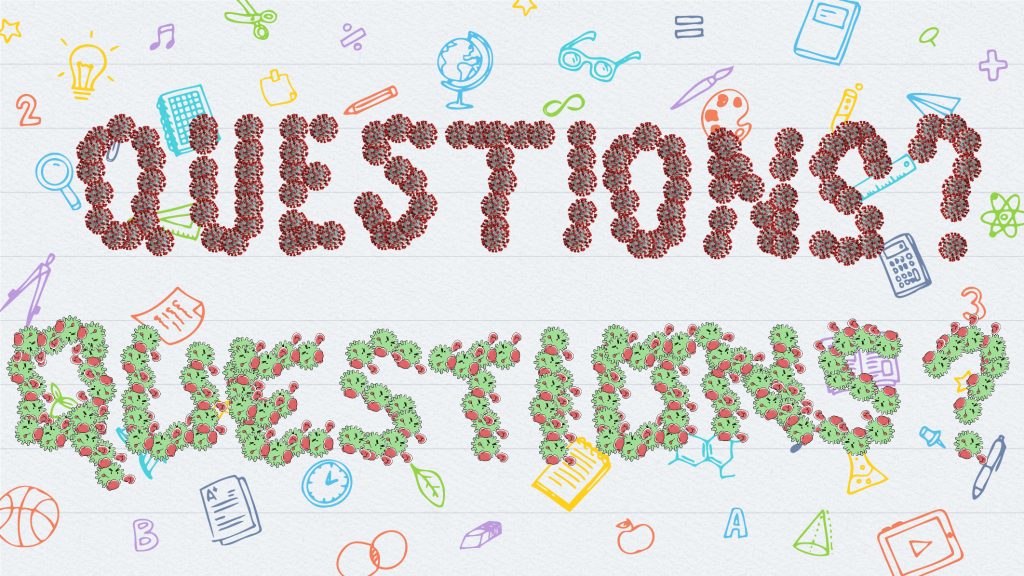